2024 Domain Adaptation with Label-Aligned Sampling (DALAS) for Cross-Domain Fault Diagnosis of Rotating Machinery under Class Imbalance
본문
- Journal
- Expert Systems with Applications
- Date
- 2024-06
- Citation Index
- SCIE (IF: 7.5, Rank: 6.1%)
- Vol./ Page
- Vol. 243, pp. 122910
- Year
- 2024
- Link
- http://doi.org/10.1016/j.eswa.2023.122910 636회 연결
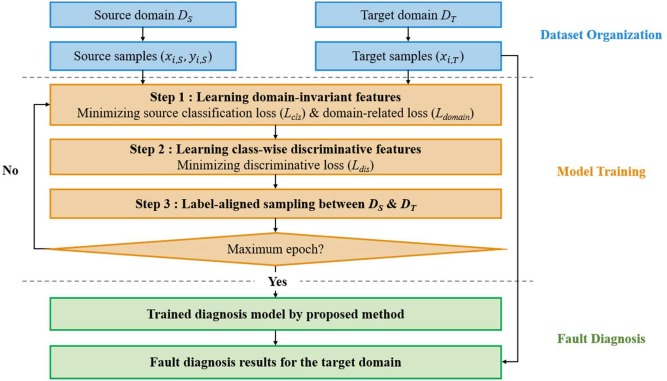
Abstract
Though existing cross-domain fault-diagnosis methods have shown promising results under domain shift conditions, existing approaches are only valid for class-balanced data. However, situations of class imbalance are inevitable in industrial fields, due to the difficulty in acquiring fault data from in-use machinery. Thus, if existing cross-domain fault diagnosis approaches are directly applied when domain shift and class imbalance coexist, performance degradation can occur. Thus, this research develops a domain adversarial learning network for class-imbalanced data to address situations where class imbalance and domain shift coexist; this situation is referred to as the problem of class imbalance domain adaptation (CIDA). In the proposed method, domain adversarial training is implemented for learning domain-invariant features by reducing the domain shift, and a label-aligned sampling strategy is utilized to deal with the class imbalance. In addition, for further performance enhancement of label-aligned sampling by increasing the accuracy of pseudo labels, metric learning is introduced to enhance the feature distinctiveness by expanding the distance of samples from different classes while decreasing the distance of samples from the same class. The efficiency of the proposed method is validated by applying it to various circumstances using two bearing datasets. The proposed method demonstrates superior performance compared to conventional algorithms in addressing the CIDA problem, according to quantitative and qualitative evaluations.