2024 PCDC: Prototype-assisted Dual-contrastive Learning with Depthwise Separable Convolutional Neural Network for Few-shot Fault Diagnosis of Permanent Magnet Synchronous Motors under New Operating Conditions
본문
- Journal
- Journal of Computational Design and Engineering
- Date
- 2024-06
- Citation Index
- SCIE (IF: 6.1, Rank: 6.6%)
- Vol./ Page
- Vol. 11, pp. 337-358
- Year
- 2024
- Link
- https://doi.org/10.1093/jcde/qwae052 717회 연결
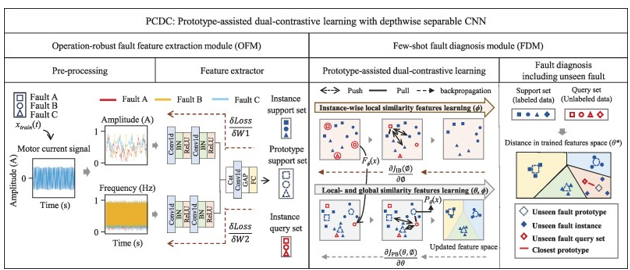
Abstract
The fault diagnosis of permanent magnet synchronous motor is of vital importance in industrial fields to ensure user safety and minimize economic losses from accidents. However, recent fault diagnosis methods, particularly the methods using deep learning, require a massive amount of labeled data, which may not be available in industrial fields. Few-shot learning has been recently applied in fault diagnosis for rotary machineries, to alleviate the data deficiency and/or to enable unseen fault diagnosis. However, two major obstacles still remain, specifically: (i) the limited ability of the models to be generalized for use under new operating conditions and (ii) insufficient discriminative features to precisely diagnose fault types. To address these limitations, this study proposes a Prototype-assisted dual-Contrastive learning with Depthwise separable Convolutional neural network (PCDC) for few-shot fault diagnosis for permanent magnet synchronous motors under new working conditions. Operation-robust fault features are extracted to reinforce generalization of PCDC under new operating conditions by extracting fault-induced amplitude and frequency modulation features and by eliminating the influence of operating conditions from the motor stator current signals. Prototype-assisted dual-contrastive learning is proposed to clearly distinguish the fault categories even when the fault features are similar to each other by learning both local- and global-similarity features, which increases the instance-discrimination ability while alleviating an overfitting issue. Experimental results show that the proposed PCDC outperforms the comparison models in few-shot fault diagnosis tasks under new operating conditions.