2024 Revolution and Peak Discrepancy-Based Domain Alignment Method for Bearing Fault Diagnosis Under Very Low-Speed Conditions
본문
- Journal
- Expert Systems with Applications
- Date
- 2024-10
- Citation Index
- SCIE (IF: 7.5, Rank: 5.2%)
- Vol./ Page
- Vol. 251, pp. 124084
- Year
- 2024
- Link
- https://doi.org/10.1016/j.eswa.2024.124084 200회 연결
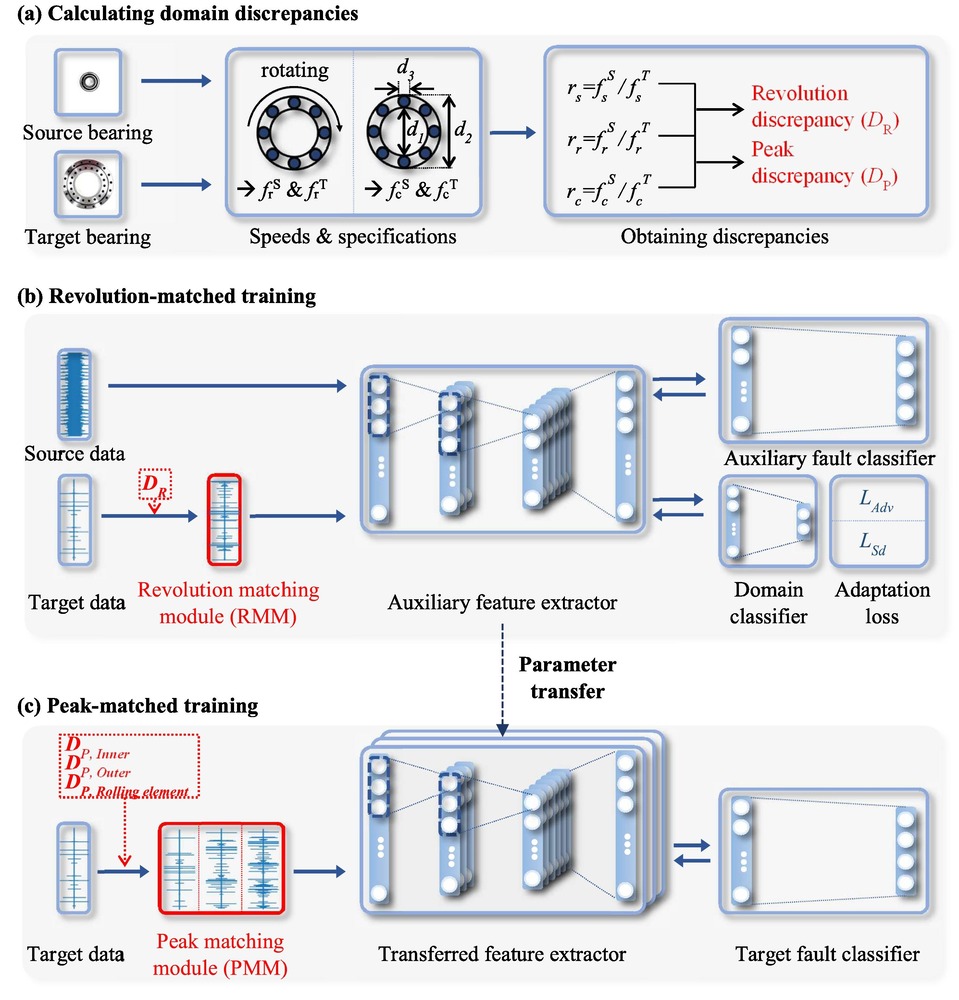
Abstract
Transfer learning (TL) for bearing fault diagnosis has achieved remarkable success under different operating conditions, even with a limited amount of data. However, it is hard to implement TL from high-speed to very-low-speed (VLS) bearings due to the large domain discrepancies. Two main factors can bring about domain discrepancies between high-speed to VLS bearings, specifically, 1) revolution discrepancy and 2) peak discrepancy that arise from different operating speeds and different bearing specifications, respectively. These factors disturb TL’s feature extraction process as it seeks speed-invariant and fault-related characteristics. Thus, this paper newly defines two metrics – revolution discrepancy DR and peak discrepancy DP – to quantify the domain discrepancies between different bearing systems, including consideration of rotational speed and specification differences. Furthermore, domain alignment techniques are proposed to reduce these domain discrepancies, thereby enabling adequate TL for bearing fault diagnosis. In this research, the revolution matching module (RMM) is developed to be helpful for extracting speed-invariant features by matching the revolution information under different rotational speeds. Further, the peak matching module (PMM) is developed to extract fault-related features by matching the defect peak information from different specifications. The proposed method is validated using experimental bearing datasets under various speeds. The results demonstrate that the proposed metrics can quantify the discrepancies between different bearing systems, and that the use of RMM and PMM can successfully improve TL performances for VLS bearings even under insufficient data conditions.