2025 Fault-relevance-based, multi-sensor information integration framework for fault diagnosis of rotating machineries
본문
- Journal
- Mechanical Systems and Signal Processing
- Date
- 2025-06
- Citation Index
- SCIE (IF: 7.9, Rank: 2.5%)
- Vol./ Page
- Vol. 232, pp. 112742
- Year
- 2025
- Link
- https://doi.org/10.1016/j.ymssp.2025.112742 26회 연결
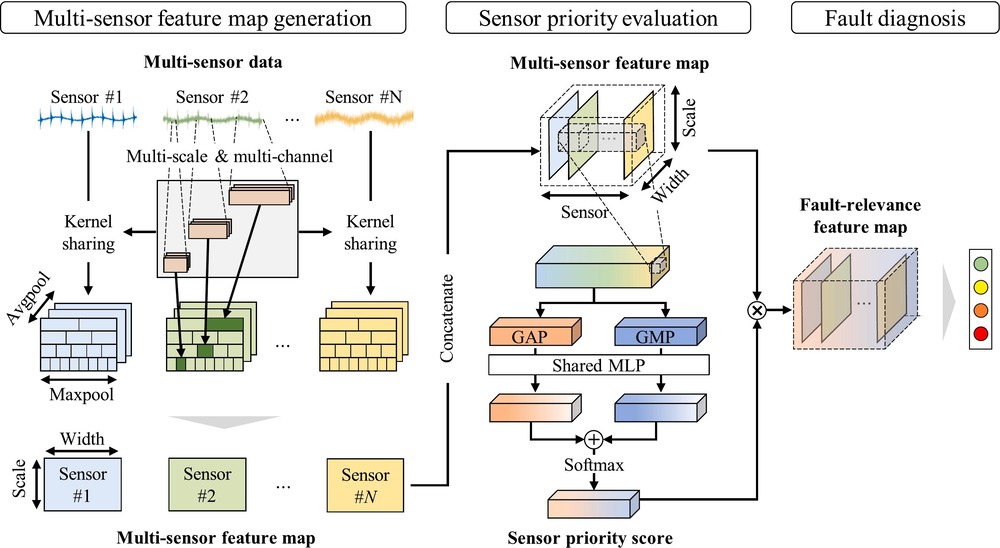
Abstract
In a multi-sensor system for fault diagnosis, each sensor’s data may contain a different amount of fault-related information, due to different transfer paths of a fault signal to each sensor. Previous research has exploited fault feature analysis to fuse multi-sensor data or simply stacked channels of data for deep learning input, entrusting the information integration task. However, existing methods are hard to identify which sensor’s data dominantly contain fault-related information, since the characteristics of all the sensors’ data gradually get mixed up as the data propagating from input to output converge through the neural network layers. Consequently, the information integration task may not scrutinize the significance of each sensor’s data (i.e., fault relevance), resulting in information loss. Therefore, this paper proposes a multi-sensor information integration framework that accounts for fault relevance of each sensor for fault diagnosis of rotating machineries. Inspired by the mechanism of a convolutional neural network (CNN), which extracts similar recurrent features in an image by the shared identical convolution kernel (the stationarity) while preserving the spatial information (the locality), a novel kernel manipulation strategy is suggested to generate a multi-sensor feature map. In the proposed method, each sensor’s data share an identical convolution kernel (the stationarity), while the unique characteristic of each sensor’s data is preserved by depth-wise convolution (the locality). Then, a sensor priority evaluation module is designed to weight fault relevance of each sensor, rather than the channel itself. Finally, an averaged attention score is multiplied by the multi-sensor feature map, thereby resulting in a fault-relevance feature map. Two experimental validation results show that the multi-sensor information is appropriately integrated proportional to the amount of the fault-related information in each sensor’s data. It is also confirmed that the proposed method outperforms existing multi-sensor fault diagnosis methods.